Digital Information Environment
59 Digital Networking – AI Algorithm Biases
Emily Rutherford
Introduction
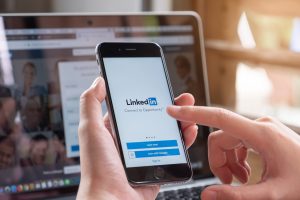
Networking and relationships are crucial for professional advancement today, and sites like Linkedin have entirely changed how individuals interact. These services recommend job opportunities, establish connections, and maximize profile visibility using Artificial Intelligence (AI) algorithms. However, bias still exists in AI programs. It frequently mirrors prevailing social injustices, which might support prejudice and disadvantage particular demographics. Systemic disparities can be impacted further by algorithmic bias, which can determine who is noticeable, hired, or overlooked in the job market. Even though AI in digital networking has opened up previously unexplored possibilities, it also raises significant issues around inclusivity, equity, and justice in professional networking settings.
AI BIAS IN NETWORKING PLATFORMS
Most digital networking sites, including LinkedIn, are powered by AI algorithms that use user data to customize networking possibilities, job suggestions, and content. However, because of the data they are educated on, AI systems frequently contain built-in biases. LinkedIn’s algorithm, for instance, has been shown to have sample bias, preferring male users in STEM disciplines or those from higher-ranking institutions disproportionately (Zhang & Vucetic, 2016). Women, minorities, and people with less prominent educational backgrounds may face obstacles as a result of this distorted exposure.
In addition, LinkedIn’s AI algorithm frequently suggests job postings based on users’ prior experiences rather than their potential or desire, according to research published in the MIT Technology Review. For those wishing to change industries or advance into higher-level roles, this may restrict their opportunities for career mobility (Metz, 2021). Another layer of prejudice developed through LinkedIn’s use of profile pictures, where users from underrepresented age or racial groups may have reduced engagement rates as a result of unconscious biases in visual perception (Magley, 2023). Such prejudices can exacerbate existing disparities and limit diverse individuals’ ability to progress or begin their careers.
INFLUENCE OF ALGORITHMS ON SELF-PRESENTATION
LinkedIn’s algorithm functions can influence users’ online personas and accounts. A University of Twente thesis claims that people frequently practice impression management by editing their profiles to seem more appealing to peers or recruiters (Lee, 2024). In order to appeal to a broader audience, this self-presentation may involve exaggerating talents, embellishing work titles, or carefully choosing profile pictures. However, the pressure to live up to algorithmic expectations might result in dishonest behavior, which will ultimately skew professional networking authenticity.
LinkedIn’s algorithm also prefers engagement-driven content, meaning posts with more likes, comments, and shares are more likely to appear in users’ feeds, according to a study by Sprout Social (Sprout Social, 2024). Users may prioritize posting viral or eye-catching posts above sharing insightful and professional expertise due to this content bias. As a result, LinkedIn’s professional value diminishes as it moves away from recognition based on talent and toward visibility driven by attractiveness.
PROFESSIONAL OPPORTUNITIES AND BARRIERS
Digital networking has opened up new career opportunities and created institutional barriers for underrepresented groups. The bias of the AI algorithm affects how profiles are visible and which individuals get considered for positions. Forbes claims that members of underrepresented racial and age groups frequently have worse exposure on LinkedIn due to the possibility that their profile images would generate unconscious biases in the algorithm, negatively impacting the likelihood of success in the job market (Magley, 2023).
Furthermore, the “network effect” makes this imbalance even worse. While those from disadvantaged backgrounds might find it challenging to get the same level of visibility, users with established professional networks are more likely to be suggested to others (Metz, 2021). This unequal prominence maintains societal injustices by limiting underrepresented communities’ access to equitable professional opportunities.
POTENTIAL SOLUTIONS
Several strategies work to reduce AI bias in digital networking. Making AI algorithms more transparent is one way to solve the problem. In order to ensure accountability and minimize bias, platforms such as LinkedIn might make their algorithmic workings publicly available. In addition, diversifying training data to include individuals from different backgrounds ensures equitable representation.
Furthermore, there has been increased support for algorithmic auditing, which examines and fixes biases in AI systems. By guaranteeing that algorithmic outputs are equitable and representative, this method can reduce the development of systemic inequities. Finally, giving users more control over their visibility through adjustable algorithm parameters could improve networking platform inclusion.
AI Acknowledgement
Artificial intelligence (AI) tools, including ChatGPT by OpenAI, were used to help generate ideas, locate and format sources, and assist with grammatical editing during the preparation of this project. All final content and critical thinking reflect my own understanding and work.
References
Lee, S. (2024). Understanding deceptive behavior on LinkedIn: The influence of psychological factors and LinkedIn usage patterns (Bachelor’s thesis). University of Twente. http://essay.utwente.nl/100666/
Magley, J. (2023, January 9). Don’t let race and age bias discourage your LinkedIn profile photo upload. Forbes.https://www.forbes.com/sites/jennifermagley/2023/01/09/dont-let-race-and-age-bias-discourage-your-linkedin-profile-photo-upload/
Metz, R. (2021, June 23). How LinkedIn’s AI bias problem feeds into a larger cycle of inequality. MIT Technology Review. https://www.technologyreview.com/2021/06/23/1026825/linkedin-ai-bias-ziprecruiter-monster-artificial-intelligence/
Ruff, L., & Frankie, J. (2020). LinkedIn: The 5-minute drill for executive networking success. Morgan James Publishing.
Sprout Social. (2024). How the LinkedIn algorithm works & optimizing your content strategy.https://sproutsocial.com/insights/linkedin-algorithm/
Zhang, S., & Vucetic, S. (2016). Sampling bias in LinkedIn: A case study. In Proceedings of the 25th International Conference Companion on World Wide Web (WWW ’16 Companion) (pp. 145–146). International World Wide Web Conferences Steering Committee. https://doi.org/10.1145/2872518.2889357
Images cited
Shutterstock. (2018, August 26). iPhone X with LinkedIn application on the screen, Chiang Mai, Thailand [Photograph]. Shutterstock. https://www.shutterstock.com/