Artificial Intelligence
77 What is Modern AI and Why it Shouldn’t be Called AI at All
Jack Hobbs
Introduction
Artificial Intelligence (AI) and Machine Learning (ML) are often used interchangeably, but they are not the same. The distinction between these two fields is critical to understanding the capabilities and limitations of current technologies. While AI aims to create systems that can perform tasks typically requiring human intelligence, ML is a subset of AI focused on enabling systems to learn from data. In today’s landscape, what is commonly referred to as “AI” is more accurately described as advanced machine learning. This chapter discusses these differences and why current technology falls short of true AI.
AI vs Machine Learning
AI, in its original conception, was envisioned as a technology that could mimic or even replicate human intelligence. Early AI research set ambitious goals, aiming to create machines that could reason, learn, and interact with the world autonomously. The Lawrence Livermore National Laboratory notes that early researchers in AI aimed to develop “machines with human-like intelligence” but quickly found this objective far more challenging than anticipated (Lawrence Livermore National Laboratory). The high hopes of achieving general AI—an intelligence that can perform a variety of tasks beyond narrow, data-driven contexts—contrast starkly with the machine learning models that dominate the field today.
Machine Learning, a subset of AI, has become the driving force behind most AI advancements today. It uses statistical techniques and algorithms to parse large datasets, identify patterns, and make predictions or decisions. According to CSU Global, much of what is considered AI today “relies on large datasets and statistical methods” rather than true cognitive capabilities (CSU Global). While these systems excel at specific, narrowly defined tasks, they lack the flexible and adaptive reasoning required for genuine AI.
The limitations of current AI technologies are clear when examined through the lens of true AI capabilities. SAS explains that today’s AI applications are essentially advanced machine learning models that “perform specific tasks by learning from data” but lack general intelligence (“Artificial Intelligence (AI): What It Is and Why It Matters”). For instance, while a machine learning algorithm can be trained to recognize images or process natural language, it does not “understand” these tasks in the human sense. It is essentially programmed to identify patterns within a dataset without any deeper comprehension or cognitive processing.
One of the primary characteristics that distinguish true AI from machine learning is the ability to understand and interact with the world. Roger Schank argues that most of what we call AI today falls short because these systems “are merely sophisticated machine learning programs” and lack true comprehension (Schank). He emphasizes that an AI capable of understanding would not only process data but would also be able to apply this information in varied, real-world scenarios with a depth of insight similar to humans. Current machine learning, by contrast, is limited to performing within the bounds of its training data.
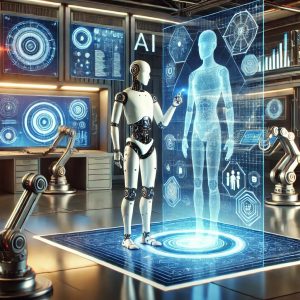
What many people believe modern AI to look like Machine learning applications are particularly prevalent in fields like advertising, where they enable targeted and personalized ads based on consumer data. Wu and Wen explain that consumers often perceive these AI-generated ads as intelligent, even though they are generated using machine learning rather than true AI (Wu and Wen). This discrepancy illustrates how advanced machine learning can mimic intelligent behavior in specific contexts, yet it lacks the versatility and adaptability that would be present in true AI.
In understanding the public’s perception of AI, it is clear that there is a gap between expectation and reality. Many individuals view AI as a technology that will one day replicate human intelligence fully. However, current machine learning models can only perform within the confines of their training, unable to generalize or adapt beyond that without further intervention. The Lawrence Livermore National Laboratory discusses the gap between the “initial goals of creating machines with human-like intelligence” and the capabilities of today’s machine learning models, reflecting the current limitations of the field (Lawrence Livermore National Laboratory). The distinction between AI and machine learning is crucial for setting realistic expectations about the future of these technologies. CSU Global points out that understanding these differences is key to recognizing that what we call AI today “is actually machine learning” (CSU Global). Such awareness can help policymakers, developers, and users make informed decisions about how to develop, regulate, and use these technologies responsibly.
Connection to STS
Conclusion
In conclusion, the differences between AI and machine learning highlight the limitations of current technology and the challenges of achieving true AI. While machine learning can simulate certain aspects of human intelligence, it does not fulfill the broader ambitions of AI research. As we continue to develop these technologies, understanding this distinction is essential for navigating both the opportunities and the limitations of what is, at its core, advanced machine learning rather than genuine artificial intelligence.
Bibliography
CSU Global. (2021, August 9). How does AI actually work? CSU Global. Retrieved from
https://csuglobal.edu/blog/how-does-ai-actually-work
SAS. (n.d.). Artificial intelligence (AI): What it is and why it matters. SAS. Retrieved from
https://www.sas.com/en_us/insights/analytics/what-is-artificial-intelligence.html
Lawrence Livermore National Laboratory. (2021, November 5). The birth of artificial
intelligence (AI) research. Retrieved from
https://st.llnl.gov/news/look-back/birth-artificial-intelligence-ai-research
Wu, L., & Wen, T. J. (2021). Understanding AI advertising from the consumer perspective.
Journal of Advertising Research, 61(2), 133-146. Retrieved from
https://www.journalofadvertisingresearch.com/content/61/2/133.short
Schank, R. C. (1991). Where’s the AI? AI Magazine, 12(4), 38. Retrieved from
https://ojs.aaai.org/aimagazine/index.php/aimagazine/article/view/917
Columbia University. (n.d.). AI vs. Machine Learning: What’s the Difference?
Columbia Engineering. Retrieved June 8, 2024, from https://ai.engineering.columbia.edu/ai-vs-machine-learning/
OpenAI. (2024). Futuristic AI scene with humanoid robot and holographic interface [Digital image].
Generated with DALL·E.
Microsoft. (2024). Copilot [AI assistant]. Retrieved from https://copilot.microsoft.com.